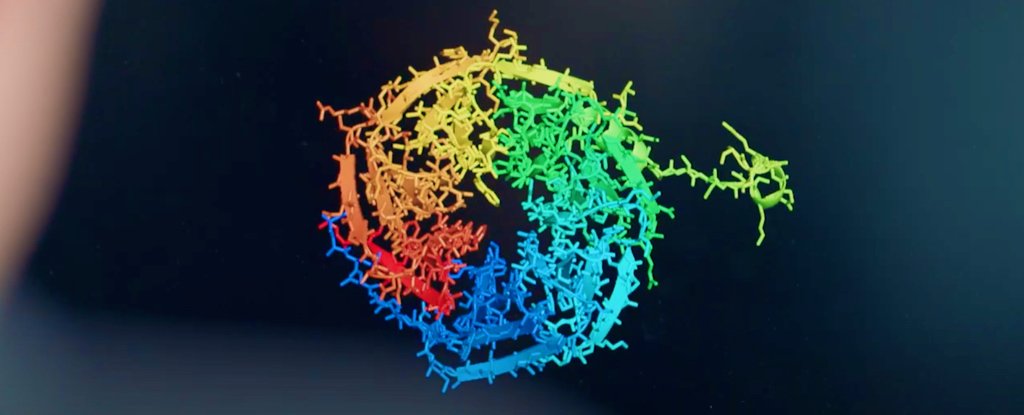
A long-standing and incredibly complex scientific problem concerning the structure and behaviour of proteins has been effectively solved by a new artificial intelligence (AI) system, scientists report.
DeepMind, the UK-based AI company, has wowed us for years with its parade of ever-advancing neural networks that continually trounce humans at complex games such as chess and Go.
All those incremental advancements were about much more than mastering recreational diversions, however.
In the background, DeepMind's researchers were seeking to coax their AIs towards solving much more fundamentally important scientific puzzles – such as finding new ways to fight disease by predicting infinitesimal but vitally important aspects of human biology.
Now, with the latest version of their AlphaFold AI engine, they seem to have actually achieved this very ambitious goal – or at least gotten us closer than scientists ever have before.
For about 50 years, researchers have strived to predict how proteins achieve their three-dimensional structure, and it's not an easy problem to solve.
The astronomical number of potential configurations is so mind-bogglingly huge, in fact, that researchers postulated it would take longer than the age of the Universe to sample all the possible molecular arrangements.
Nonetheless, if we can solve this puzzle – known as the protein-folding problem – it would constitute a giant breakthrough in scientific capabilities, vastly accelerating research endeavours in things like drug discovery and modelling disease, and also leading to new applications far beyond health.
For that reason, despite the scale of the challenge, for decades researchers have been collaborating to make gains in developing solutions to the protein-folding problem.
A rigorous experiment called CASP (Critical Assessment of protein Structure Prediction) began in the 1990s, challenging scientists to devise systems capable of predicting the esoteric enigmas of protein folding.
Now, in its third decade, the CASP experiment looks to have produced its most promising solution yet – with DeepMind's AlphaFold delivering predictions of 3D protein structures with unprecedented accuracy.
"We have been stuck on this one problem – how do proteins fold up – for nearly 50 years," says CASP co-founder John Moult from the University of Maryland.
"To see DeepMind produce a solution for this, having worked personally on this problem for so long and after so many stops and starts wondering if we'd ever get there, is a very special moment."
In the experiment, DeepMind used a new deep learning architecture for AlphaFold that was able to interpret and compute the 'spatial graph' of 3D proteins, predicting the molecular structure underpinning their folded configuration.
The system, which was trained up by analysing a databank of approximately 170,000 protein structures, brought its unique skillset to this year's CASP challenge, called CASP14, achieving a median score in its predictions of 92.4 GDT (Global Distance Test).
That's above the ~90 GDT threshold that's generally considered to be competitive with the same results obtained via experimental methods, and DeepMind says its predictions are only off by about 1.6 angstroms on average (about the width of an atom).
"I nearly fell off my chair when I saw these results," says genomics researcher Ewan Birney from the European Molecular Biology Laboratory.
"I know how rigorous CASP is – it basically ensures that computational modelling must perform on the challenging task of ab initio protein folding. It was humbling to see that these models could do that so accurately. There will be many aspects to understand but this is a huge advance for science."
It's worth noting that the research has not yet been peer-reviewed, nor published in a scientific journal (although DeepMind's researchers say that's on the way).
Even so, experts who are familiar with the field are already recognising and applauding the breakthrough, even if the full report and detailed results are yet to be seen.
"This computational work represents a stunning advance on the protein-folding problem, a 50-year old grand challenge in biology," says structural biologist Venki Ramakrishnan, president of the Royal Society.
"It has occurred decades before many people in the field would have predicted."
The full findings are not yet published, but you can see the abstract for the research, "High Accuracy Protein Structure Prediction Using Deep Learning", here, and find more information on CASP14 here.
https://news.google.com/__i/rss/rd/articles/CBMia2h0dHBzOi8vd3d3LnNjaWVuY2VhbGVydC5jb20vYWktc29sdmVzLTUwLXllYXItb2xkLWJpb2xvZ3ktZ3JhbmQtY2hhbGxlbmdlLWRlY2FkZXMtYmVmb3JlLWV4cGVydHMtcHJlZGljdGVk0gFvaHR0cHM6Ly93d3cuc2NpZW5jZWFsZXJ0LmNvbS9haS1zb2x2ZXMtNTAteWVhci1vbGQtYmlvbG9neS1ncmFuZC1jaGFsbGVuZ2UtZGVjYWRlcy1iZWZvcmUtZXhwZXJ0cy1wcmVkaWN0ZWQvYW1w?oc=5
2020-12-01 02:03:06Z
52781217477573